Pedestrian Detection in Thermal (Infrared) Images Sample Paper
Pedestrian Detection in Thermal (Infrared) Images
Late advances in science and innovation have empowered the development of tracking machines to cooperate with individuals. This has made a monstrous chance for vision information designing and examination to assume a fundamental part in a wide scope of utilizations from non-military personnel safe heading to public security. Identifying individuals in pictures is a basic exploration of human conduct examination. However,it assumes a principal part in cutting edge driver collaborator framework (ADAS) and self-ruling driving, particularly with the development of maturing populace (Goubet et al., 2006). For cutting edge driver associate frameworks, passerby identification means to dodge crashes and mishaps by offering advancements that ready drivers the possible perils or executing shields by starting defensive measures, such as assuming responsibility for the vehicles. Because of vehicles' development spray, auto collisions possess huge fatalities, with walkers being the weakest traffic members.
Agents uncovered that more than 10 million individuals experienced car crashes around the globe consistently, and 2–3 million of those individuals were genuinely harmed. Besides, walkers represent 24% of all traffic fatalities worldwide, and passerby mishaps have the most noteworthy casualty rate among all traffic member gatherings, with about 8% of all common mishaps being lethal. By and large, walkers are distinguished from dark scale or shading pictures. Be that as it may, human bodies have the quality of inflexibility and adaptability, and there is a wide scope of conceivable passerby appearance because of enlightenment, impediment, verbalized posture, and complex foundation (Goubet et al., 2006). Once in a while, the utilization of obvious pictures isn't possible without outer counterfeit light, particularly at evening time. Along these lines, walker location is a troublesome undertaking. Conversely, warm pictures are caught by warm sensors that sense transmitted radiation from objects of interest, for example, people or vehicles. Warm pictures utilize the power maps, which give an upgraded phantom reach to make people notice and feature the differentiation between objects of the high-temperature difference and the cool foundation.
In this way, it is conceivable to distinguish people on foot from warm pictures with deficient or over enlightenment. Also, the inconstancy presented by shading, surface, and complex foundation gets paltry. Walker discovery is regularly ordered as a 2-classes object characterization issue, where learning strategies are utilized to arrange a competitor article or district into a person on foot or non-pedestrian. The walker location system, commonly, comprises the accompanying advances: applicant determination, highlight extraction, and highlight order. For walker identification with warm pictures, specialists misused the warm difference in the pictures to distinguish the likely places of competitor people on foot (Goubet et al., 2006). Discriminative highlights are extricated and given as contributing to the grouping structure from the competitor people on foot. The delegation boundaries are produced for the picked classifier because of the adequate highlights from earlier known walkers and non-people on foot.
At that point, the classifier is utilized to join names to the applicants' highlights on foot. Critical consideration has been paid to removing discriminative highlights that improve the passerby location rate while diminishing the bogus positive rate. In work by Olemda et al., to diminish the impact brought about by the light change in warm pictures, picture stage congruency include was utilized to identify the people on foot. Gawande et al. (2020)arranged the applicants utilizing multidimensional histograms, dormancy, and differentiation-based highlights. The competitors were chosen utilizing a projection-based division technique. As of late, Gawande et al. (2020) proposed a component descriptor named pyramid entropy weighted histograms of arranged slopes to address the up-and-comer walkers.
The applicant people on foot were recognized utilizing warm angle and limit-based picture division. While discriminative highlights essentially improve the recognition exactness, foundation clamor is a test for the person on foot discovery. In Davis et al., a two-stage layout-based technique is proposed to find applicant passerby areas utilizing shape saliency maps. The guessed individual areas are approved with an AdaBoost gathering classifier with a consequently tuned channel (Ko, 2011). Wang et al. broadened this methodology by fusing the following inside their structure. Up-and-comer walkers removed by warm force data are characterized utilizing a learned help vector relapse model. A passerby tracker was utilized to restrict the recognized walkers and adaptively update the identification boundaries. The versatile structure was appeared to build the heartiness of the passerby location. A comparable methodology, where recognition and following were all the while actualized.
Movement and appearance-based highlights were utilized to fit as fiddle data inside an EM-based structure to identify the people on foot. Multimodal data combination was likewise received to build the person's heartiness on foot identification in fluctuating light conditions. Davis et al. joined data from warm and obvious pictures to perform competitor choice, highlight extraction, and highlight arrangement utilizing person-on-foot shape data. Shape saliency maps were produced to feature notable items with the information and foundation angle data. People on foot forms were then separated from the created saliency maps (Gawande, 2020). Likewise, Ge et al. consolidated noticeable and warm pictures inside a synchronous walker recognition and following structure. To represent foundation clamor and light varieties, the creators prepared numerous classifiers on disjoint subsets of various picture sizes. The prepared classifiers were then orchestrated in a tree design to perform location in a coarse-to-fine way.
The meager portrayal has recently discovered different picture handling uses, such as face acknowledgment, picture rebuilding, picture similitude evaluation, and hyperspectral picture arrangement. One of its launches, the least portrayal length standard, specifies that helpful data that yields the most minimal portrayal should be liked for explicit dynamic assignments, such as characterization, that yields the most minimal portrayal in high dimensional information (Gawande, 2020). In scanty portrayal, the inadequate word reference is made out of premise particles, which are misused as highlight vectors from earlier realized walker and non-person on foot preparing tests. In high dimensional space, a meager portrayal looks to show complex information with a subset of premise molecules.
Inadequate coefficients are figured from an overcomplete word reference (for example, the quantity of premise iotas surpasses the element of the sign) by taking care of the improvement issue concerning a sparsity limitation. At that point, the information viable can be roughly addressed with a direct blend of those picked premise molecules and related scanty coefficients (Ko, 2011). The fundamental aim of inadequate portrayal is to reproduce the info signal by a conservative portrayal. This methodology is ordinarily alluded to as molecule disintegration and is prepared with pursuit calculation via looking through the conceivable guess. A few productive pursuit calculations have been proposed (Xu et al., 2005). Symmetrical coordinating pursuit (OMP) is proposed as an adjusted ravenous calculation of coordinating pursuit (MP).
One of the main tasks in video-based surveillance is identifyingpassersby in a series of video shots confining everyone. The problem correlates with determining the regions. A region is the smallest bounding rectangle found in the video series that confines all people. A majority of the structures use the people's positions, any previous visits to the scenes, and study the trajectories to recognize human habits (Xu et al., 2005). Existing literature outlines a mixed approach of body tracking, figure examination and also creates how people look. With the use of a single camera, this is a good design for an outdoor environment. Even in instances where there is partial obstruction, the structure discerns and tracks human beings and evaluates their behavior. The detected paths form the basis for optimal performance for the systems.However, in some cases, the outcomes are inadequate for identifying vigoroushuman tasks and incident evaluation (Xu et al., 2005). The modern video monitoring systems comprise several features like motion detection, the study of human behavior, detecting, and trailing.
Motion and Object Detection
Object and motion detection is the initial step that manages to identify examples of semantic objects of a specific class, for example, people, structures, vehicles, and so on, in a succession of recordings. The various article recognition methodologies are outline-to-outline distinction, foundation deduction, and movement investigation utilizing optical stream methods (Wang et al., 2003). These methodologies regularly utilize removed highlights and learning calculations to perceive occurrences of an item class. The article location measure is separated into two classes. First, object location incorporates fundamentally three kinds of strategies, for example, foundation deduction, optical stream, and spatiotemporal separating. Second, object grouping utilizes principally visual highlights as shape-based, movement-based, and surface-based techniques. Movement discovery is one of the issues in video reconnaissance. It isn't just liable for the extraction of moving items, yet also basic to numerous applications, including object-based video encoding, human movement examination, and human-machine associations(Wang et al., 2003). After item location, the following stage is movement division. This progression is utilized for recognizing locales comparing to moving articles, for example, people or vehicles. It mostly centers around identifying moving locales from video outlines and making a data set for following and conduct an investigation. Movement recognition is utilized for identifying a change in the situation of an item, comparative with its environmental factors or an adjustment in the environmental factors, comparative with an item (Khandhediya et al., 2017). Movement identification can be accomplished utilizing electronic movement sensors, which distinguish the movement from the genuine climate.
Object Tracking
Following items in a video arrangement implies recognizing a similar article in a grouping of edges utilizing the novel article qualities addressed in the structure of highlights. For the most part, the discovery interaction is constantly trailed by following in video observation frameworks. Following is performed starting with one edge then onto the next, utilizing the following calculations, for example, portion based following, point-based following, and outline based following (Khandhediya et al., 2017).
Activity and Behavior Analysis
In certain conditions, it is required to examine the practices of individuals and decide if their practices are dubious or not, for example, the conduct of a person on foot at a packed spot (for example, public, commercial centers, and government workplaces, and so forth) In this progression the movement of items is perceived from the video scene and create the depiction of the activity. Dai et al. (2007) proposed a basic examination and displaying technique of human groups to choose the most important scale-out of three methodologies, i.e., (1) minute, implies walker are individual recognized dependent on the area, speed, and movement boundary is ignored, (2) mesoscopic, implies passerby are distinguished dependent on position, speed, what's more, rely upon the dispersion capacity and (3) perceptible, mean the walker are distinguished depending on the normal walker amount, snapshot of a walker. It may be utilized for productive dynamic in basic circumstances when Ongoing Trends in Computational Intelligence human group security is significant. Human groups' well-being relies on the amount and thickness of person on foot move actually at various high crowed places.
Person Identification
The last advance is recognizable human proof. Human face and step are the primary biometric highlights utilized for individual recognizable proof in visual observation frameworks after a conduct examination(Dai et al., 2007). This part aims to talk about the issues and difficulties associated with planning a visual reconnaissance framework. Once more, bunch passerby recognition and following techniques utilized for moving and fixed camera into general classifications and give an educational investigation of relative strategies in every class. The primary commitments of this section are as per the following:
• The similar examination of freely accessible benchmark datasets of the person on foot with its utilization, determination, and climate constraint.
• Analyze issues and difficulties of walker identification and following in the video groupings caught by a moving and fixed camera.
• Categorizing the strategies for passerby identification and following various ways depends on the overall idea of techniques having a place with every class and depicted proposed enhancements for every strategy.
Recent Trends in Computational Intelligence
Tracking human beings is a challenging task since different people have different shapes and appearances. This is largely due to varied visual perspectives. Some scholars came up with a control structure of dynamic cameras used in a multiple-camera video surveillance structure. Therefore, most research works focus on active multiple- camera people detection instead of fixed camera people detection. Pedestrian Tracking pedestrians have been carried out by staticcameras employing a figure-based technique, which discerns and collates shapes of people in successive frames. As described in, cameras are graded using a similarscene-wide mark coordinate structure. Scholars designed a structure used in tracking head and facial parts of people through
A tiered tracking technique using a PTZ camera and a fixed camera. The modern surveillance system centers on tracking people by detection, as shown in. He merged the first estimate of the human positions across the builds in a tracking by-detection structure mixed points of body parts across and within the frames from a package of tractable subunits. Suggested employing a combination of body parts for detecting and tracking partially blocked people.
Tracking people is a more challenging task when using moving cameras than in fixed cameras. Most successful human tracking methods used in a fixed camera, like modeling, a regular ground plane assumption, and background subtraction, make the process more challenging. Human detectors are largely applied to detect people in the videos instead of applying background design techniques to get human behavior. Therefore, the daunting task is to successfully spot the people rotating cameras and later use them to track people. Even though people detectors may successfully extract people, they still have limitations. Human detectors sometimes give erroneous signals or completely miss human detection when they are either fully or incompletely blocked. In such situations, human detections fail, and spotting them becomes erratic until when the person reappears under the frames. It is shown that there is a lot of research work on the numerous hindrances of spotting and training people.
However, there still lacks completely reliable solutions to the hindrances, as shown above. Most of the designs of humandetection and tracking were evaluated in both enclosed and outside environments. Several trials were also carried out to approximate the system's accuracy in detection rate, speed, and complexity (Negied et al., 2015). The researchers' analysis of designs shows that in-depth learning models of human detection and tracking processes can succeedin real-time situations. However, there is ample space for improving the existing methods of human detection and tracking in video surveillance systems.
Challenges in Video Acquisition
Illumination Variation
There are several lighting conditions of the location. There is a probability the target might move due to the source of light, various times of the day, reflection from shiny surfaces, outdoor or indoor sites, and partial or full obstruction of the light source. These factors' direct effect results in changes in the background appearance, which causes wrong positive detections for the techniques based on background modeling(Dai et al., 2007). Therefore, these techniques need to adjust the models to the slight variations. And since the object's appearance varies depending on the lighting differences, appearance-based tracking techniques may fail to trail the pattern's target object. Therefore, it is fundamental for these techniques to apply features that do not change depending on lighting variation.
Presence of Abrupt Motion
Abrupt changes in direction and speed of the object's movement or abrupt camera movement are another limitation of video capturing that impacts spotting and tracking the object.
Recent Trends in Computational Intelligence
Differencing techniques may not spot the parts of the item logical to the background. Meanwhile, a quick movement brings forth a line of the blurred spotted area. If this object's movements or camera movements are not reviewed, the item cannot be spotted well by techniques premised on background designs(Dai et al., 2007). Simultaneously, the tracking-based techniques and projection of movement become quite hard and sometimes become impossible. When this happens, the tracker loses the target. Still, even when the tracker does not entirely lose it, the unprojected movement can result in a higher amount of flaw in the designs.
Complex background
In natural outdoor scenes, there is a high variation of textures. Furthermore, the background can be active, i.e., like it may have moved. The examples include a water fountain, moving clouds, water waves, tides, changing traffic lights, and swaying trees. These movements need to be taken into consideration as they may alter object detection designs. It is important to remember that the motions can either be regular or irregular.
Shadows
Obstruction of the object by the source of light creates shadows. The existence of shadows in video series hardens the task of detecting a moving item. A shadow is considered static if there is no object motion in the sequence. The resulting shadow can be successfully absorbed into the framework. However, an active shadow, caused by an object in motion, has a critical effect on accurately spotting an object in motion. The moving object has similar motion characteristics and is joined to it. If we analyze the observed features like texture, colors, and edges, it is possible to remove the shadow from the sequence's image (Nanda & Davis, 2002). We can also use a model premised on prior data like lighting conditions and the object's shape in motion. However, active shadows still pose a challenge in differentiating them from moving objects. It is extremely difficult for outside scenes where the backdrop is usually complicated.
Challenges in human detection and tracking
Pedestrian Obstruction
Other objects on the site may also block the object. Somebody parts can be slightly concealed behind the other items (partial obstruction). And in scenario two, the object is fully concealed by the other objects (complete obstruction). For example, take the target object to be a person walking on the walkway. The target may be blocked by shrubs and flowers on the sidewalk, vehicles in the street, and other people on the sidewalk (Negied et al., 2015). Obstruction seriously impacts objects' detection using background design techniques, where the item is absent or set apart into other disjointed scenes. If an obstruction occurs, the item's outward form can change briefly, which can cause challenges in applying some of the object tracking techniques.
Pose Variation: Moving Item Outward Form Changes
In actual schemes, most items can take in three- dimensional scope, but we get the projectionof their three- dimensional motion in a two- dimensional position. Therefore, spinning in the third axis's direction may cause the item's outward form to vary. Variation in posture impacts the tracking design performance. Similar people look different in successive frames if there are continuous posture changes (Negied et al., 2015). It is also for the objects to change their appearance. All these can be done by changing clothes, rocking different hairstyles, donning a headscarf, etc. Over a while, a target's appearance may change, especially for a nonrigid object. The main aim is tracking people, which becomes difficult under these circumstances.
References
Dai, C., Zheng, Y., & Li, X. (2007). Pedestrian detection and tracking in infrared imagery using shape and appearance. Computer Vision and Image Understanding, 106(2-3), 288-299.
Gawande, U., Hajari, K., &Golhar, Y. (2020). Pedestrian Detection and Tracking in Video Surveillance System: Issues, Comprehensive Review, and Challenges. Recent Trends in Computational Intelligence.
Goubet, E., Katz, J., &Porikli, F. (2006, May). Pedestrian tracking using thermal infrared imaging. In Infrared technology and applications XXXII (Vol. 6206, p. 62062C). International Society for Optics and Photonics.
Khandhediya, Y., Sav, K., &Gajjar, V. (2017). Human detection for night surveillance using adaptive background subtracted image. arXiv preprint arXiv:1709.09389.
Ko, T. (2011). A survey on behaviour analysis in video surveillance applications. Video Surveillance, 280-293.
Nanda, H., & Davis, L. (2002, June). Probabilistic template based pedestrian detection in infrared videos. In Intelligent Vehicle Symposium, 2002. IEEE (Vol. 1, pp. 15-20). IEEE.
Negied, N. K., Hemayed, E. E., &Fayek, M. B. (2015). Pedestrians' detection in thermal bands–Critical survey. Journal of Electrical Systems and Information Technology, 2(2), 141-148.
Wang, L., Hu, W., & Tan, T. (2003). Recent developments in human motion analysis. Pattern recognition, 36(3), 585-601.
Xu, F., Liu, X., & Fujimura, K. (2005). Pedestrian detection and tracking with night vision. IEEE Transactions on Intelligent Transportation Systems, 6(1), 63-71.
Popular Blog Posts
Personal Experiences of an Immigrant Student
This paper explores pers...
Story Map Picture Book
Story Map Picture Book Intr...
Climate Change Perspectives at KPU
Climate Change Perspectives at...
Communication Analysis Assignment - GM & Netflix (Why Not An EV?)
Instructions - Communica...
Beauty Industries and Black Women
In African A...
Sociological Perspectives || Functionalism as a Tool for Social Change
GSOC 101: DISCUSSION 1 ...
ELM 570 Topic 6 Discussion 1 & 2 || Technologies That Facilitate Science Instruction & Making Science Instruction Engaging For Students
TABL...
ELM 570 Topic 5 Discussion 1 & 2 || Summative Vs Formative Assessments and Assessment Processes
TABLE OF CONTENT...
ELM 570 Topic 4 Discussion 1&2 || How Can Students Access Technology and Incorporate it in Science Instruction and Developmental Levels
TABLE OF C...
Odyssey Essay :- Theme on Loyalty
THEME: Loyalty ...
Place Order
100% Moneyback
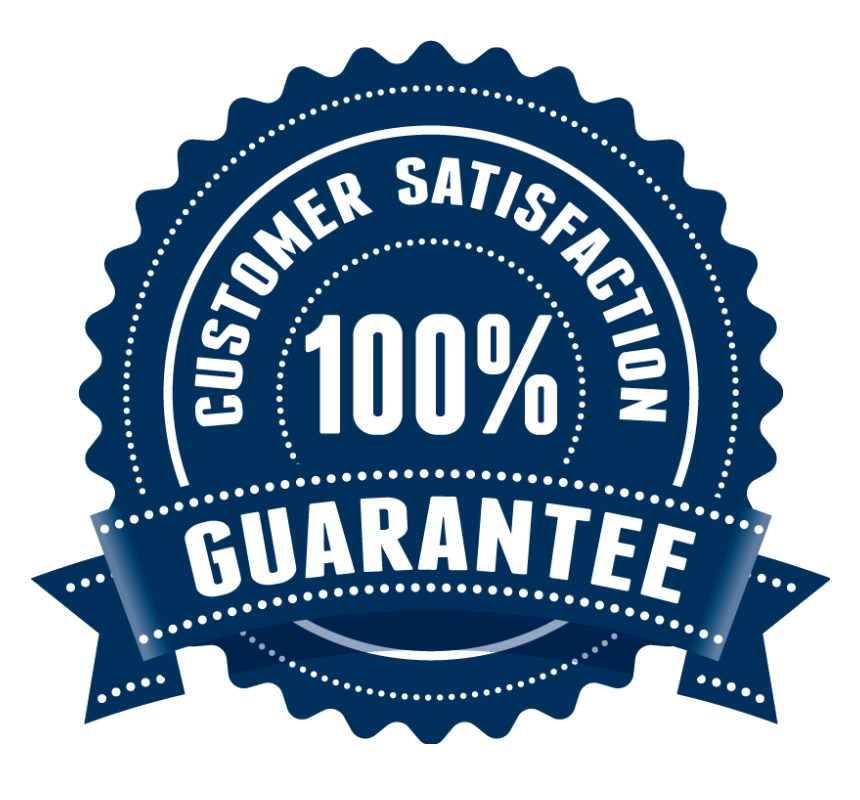